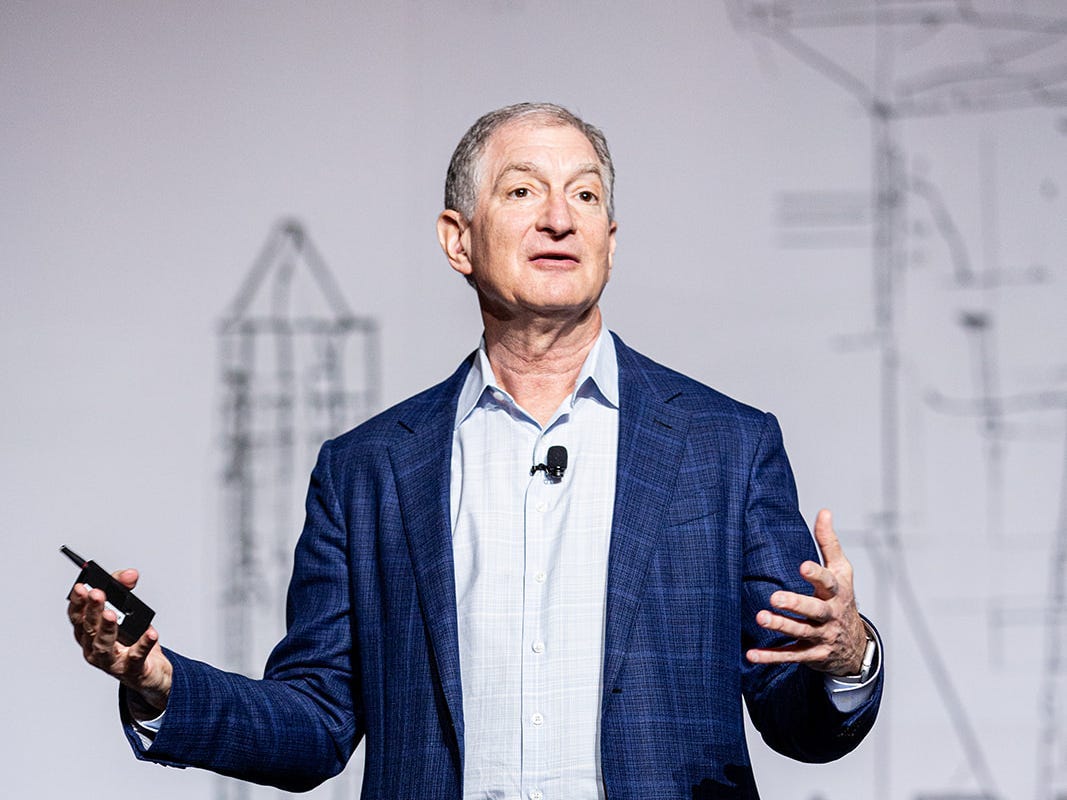
2025 ARPA-E Energy Innovation Summit
The lion’s share of artificial intelligence workloads moving from training to inference is great news for AMD, its CTO said.
AI training workloads — the ones that make up the gargantuan task of building large language models, imbuing them with a familiar writing or speaking style, and knowledge — used to be most of what AI computing was used for. Inference is the computing process that happens when AI generates outputs like answering questions or creating images.
It’s hard to pin down exactly when the switch happened — probably some time last year. But inference is now and will likely stay the largest segment of accelerated computing going forward. Since then, AMD executives have been hyping up a window of opportunity to wrest market share from Nvidia.
“People like the work that we’ve done in inference,” CEO Lisa Su said on the company’s February earnings call.
AI at scale is all about inference.
If you ask Mark Papermaster, AMD’s Chief Technology Officer, where it all goes from there, he’ll tell you that as inference grows, it’s headed for the edge.
“Edge devices” are the industry term for computers that live outside the data center. Our phones and laptops all qualify, but so could smart traffic lights or sensors in factories. Papermaster’s job is to make sure AMD is headed in the right direction to meet the demand for AI computing across devices as it grows.
AMD has had to play catch-up in the data center since Nvidia’s 10-year head start. But at the edge? The field is more open.
Business Insider asked Papermaster what he thinks the future of handheld AI looks like.
This Q&A has been edited for clarity and length.
What’s the most prominent use for AI computing in edge devices like laptops and phones?
The use case you’re starting to see is local, immediate, low-latency content creation.
Why do we use PCs? We use them to communicate, and we use them to create content. As you and I are talking — this is a Microsoft Teams event — AI is running underneath this. I could have a correction on it such that if I look side to side, you just see me centered. That’s an option. I can hit automatic translation — you could be in Saudi Arabia and not speak any English, and we could have simultaneous translation once these things become truly embedded and operational, which is imminent.
It’s truly amazing what’s coming because just locally on your PC, you’ll be able to verbally describe: ‘Hey, I’m building a PowerPoint. I need this. I need these features. I’m running Adobe. This is what I want.’
Today, I’ve got to go back to the cloud. I’ve got to run the big, heavy compute. It’s more expensive and it takes more time.
That’s the immediate example that’s front and center, and this is why we’ve invested heavily in AI PCs. That’s imminent from Microsoft and others in the next six months.
The other application that we’re already seeing is autonomous anything. It starts with cars, but it’s way beyond cars. It’s the autonomous factory floor.
OK, say it’s 2030 — how much inference is done at the edge?
Over time, it’ll be a majority. I can’t say when the switch over is because it’s driven by the applications — the development of the killer apps that can run on edge devices. We’re just seeing the tip of the spear now, but I think this moves rapidly.
You might consider phones as an analogy. Those phones were just a nice assist until the App Store came out and made it really easy to create a ton of applications on your phone.
Now, things that used to always be done with more performant computing could be done more locally. Things that were done in the cloud could be done locally. As we start to get killer applications, we’re going to start to see that shift go very rapidly. So it’s in the next three to six years, no doubt.
I keep running into examples that suggest the way models are getting better is to just keep piling on more inference compute.
How do you know that three years from now, there’s not going to be some breakthrough that makes all these devices being designed now completely out of date?
Everything you’re describing is to gain even more capability and accuracy. It doesn’t mean that what we have is not useful. It’s just going to be constantly improving, and the improvement goes into two vectors.
One vector is becoming more accurate. It can do more things, and typically drives more compute. There’s an equal vector that runs in parallel, saying, ‘How could I be more optimized?’
I call it the DeepSeek moment. It sort of shook the world. Now you have everybody — Microsoft, Meta, Google — making their models more efficient. So you have both examples where it’s taking more and more compute and examples where there’s innovation driving more and more efficiency. That’s not going to change.
The post AMD’s CTO says AI inference will move out of data centers and increasingly to phones and laptops appeared first on Business Insider.